Unlocking the Power of Video Annotation Tools for Machine Learning
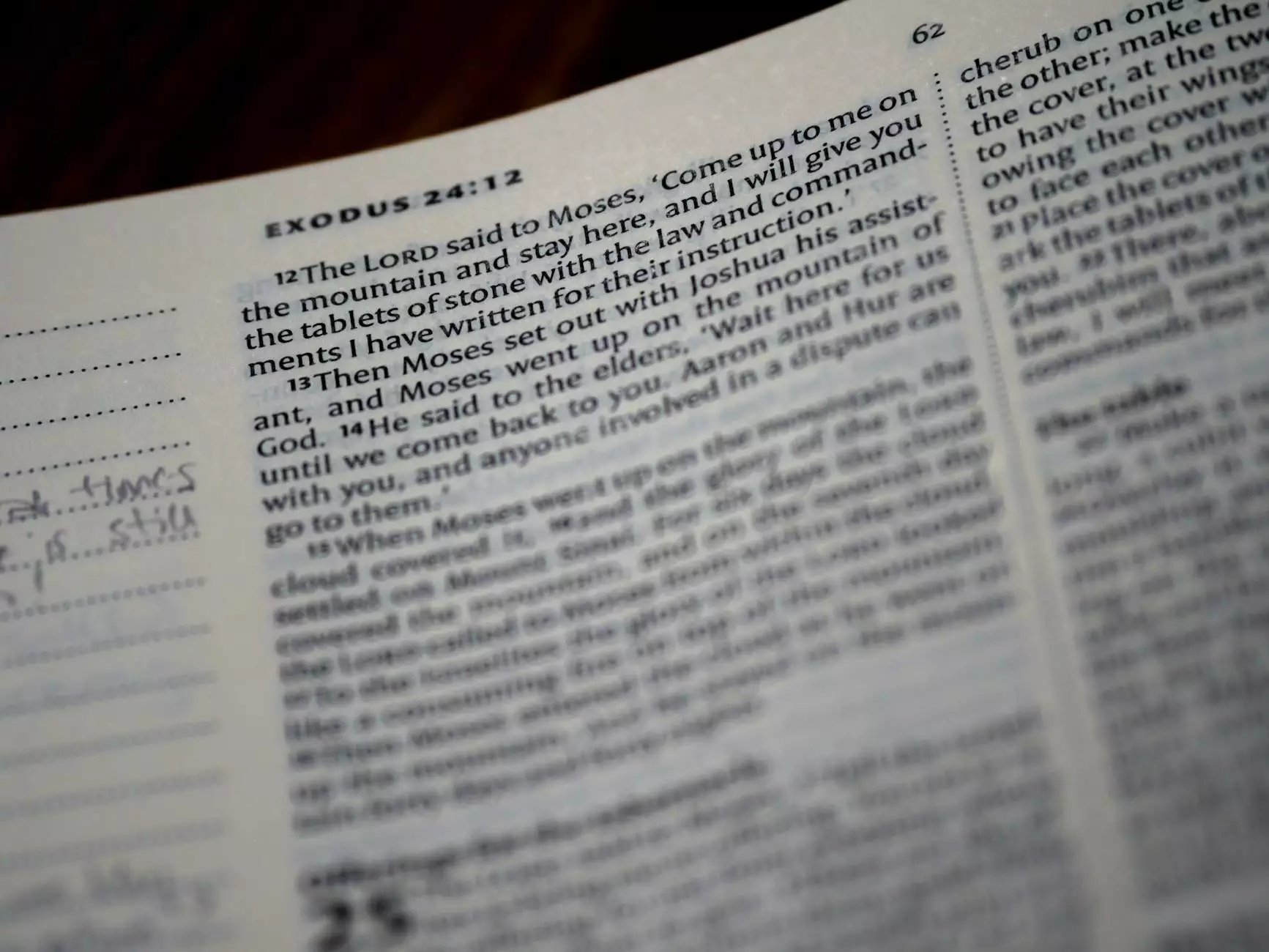
In the rapidly evolving domain of machine learning, the ability to utilize high-quality training data is paramount. Video annotation tools for machine learning have emerged as essential components in creating the datasets needed for developing robust AI models. This article delves deep into the world of video annotation, exploring the functionalities, benefits, and significance of these tools, particularly in the realm of machine learning within the context of KeyLabs.ai.
Understanding Video Annotation
Video annotation refers to the process of labeling video frames with relevant information to help machine learning models learn to recognize patterns, objects, and actions within the video content. This intricate process is vital for various applications ranging from autonomous vehicles to security surveillance systems.
Types of Video Annotations
Video annotations can take various forms, making them adaptable to different machine learning tasks. The main types include:
- Object Detection: Labeling specific objects within video frames, helping the AI recognize and track them over time.
- Semantic Segmentation: This involves assigning a class label to every pixel in the frame, providing a more detailed understanding of scene components.
- Action Recognition: Annotating sequences of actions depicted in the video, crucial for understanding activities in surveillance or sports analytics.
- Tracking: Continuously annotating the position of objects across frames, vital for dynamic environments.
The Importance of Video Annotation Tools
Video annotation tools are indispensable for any project involving machine learning, catering to a range of industries and use cases. Here are several critical reasons to embrace these tools:
1. Enhancing Model Accuracy
Machine learning models learn from labeled data. The quality of annotations directly affects the model's performance. By leveraging effective video annotation tools, organizations can ensure their data is annotated consistently and accurately, leading to better model predictions.
2. Accelerating Development Cycles
Manual video annotation can be tedious and time-consuming. By utilizing a dedicated video annotation tool, organizations can streamline the annotation process, significantly reducing the time taken to make training data ready. This efficiency allows data scientists and machine learning engineers to focus on model development rather than data preparation.
3. Facilitating Collaboration
Many video annotation tools offer collaborative features that enable teams of annotators to work together. This is especially useful for large projects, ensuring that all team members can contribute effectively while maintaining a consistent annotation style.
4. Supporting Diverse Use Cases
From medical imaging and autonomous driving to video surveillance and consumer behavior analysis, video annotation tools can be applied across various industries. This versatility makes them a valuable investment for organizations looking to enhance their machine learning capabilities.
Key Features of Video Annotation Tools
When selecting a video annotation tool, it is essential to look for features that optimize the annotation process and enhance the quality of the output. Below are several key features to consider:
1. Intuitive User Interface
A user-friendly interface allows annotators to navigate the tool effortlessly, minimizing the learning curve and enabling quicker onboarding for new team members.
2. Advanced Annotation Techniques
Look for tools that offer a variety of annotation methods, such as bounding boxes, polygons, and keypoint annotation. These features support a wide range of applications and improve the precision of the annotations.
3. Integration with Machine Learning Frameworks
Choose video annotation tools that can easily integrate with popular machine learning frameworks such as TensorFlow and PyTorch. This compatibility ensures that your annotation output can directly feed into your training pipelines, streamlining the overall workflow.
4. Quality Control Mechanisms
Tools that include built-in review systems allow for quality checks and feedback on annotations, ensuring that the labeled data meets the necessary quality standards before being used for training models.
5. Cloud-Based Solutions
Cloud-based video annotation platforms allow annotators to work from anywhere, foster collaboration, and provide easy access to large video datasets without the need for extensive local hardware.
Applications of Video Annotation Tools for Machine Learning
The applications of video annotation tools are broad and varied, fundamentally altering how industries utilize machine learning. Below are several key industries and their respective applications:
1. Autonomous Vehicles
In the automotive industry, video annotation is utilized to train algorithms for recognizing pedestrians, other vehicles, traffic signals, and various obstacles. Accurate annotations are crucial for the safety and reliability of self-driving technology.
2. Surveillance and Security
For surveillance systems, video annotation tools help in identifying suspicious activities or behaviors. By tagging relevant events in video feeds, these tools enable quicker responses and enhance situational awareness.
3. Healthcare
In healthcare, video annotation can be used to analyze medical imaging or video demonstrations of surgical procedures. Annotated videos can assist in training models that support diagnostics or enhance surgical training.
4. Sports Analytics
Sports organizations use video annotation to analyze player movements, strategies, and game outcomes. By annotating game footage, teams can gain insights into performance and develop training programs that address weaknesses.
5. Consumer Behavior Analysis
Retailers can analyze video feeds from in-store cameras to understand customer behaviors better. Video annotation tools aid in identifying patterns in movement, interactions, and product selections, providing valuable insights for marketing and layout strategies.
Choosing the Right Video Annotation Tool for Your Needs
With a plethora of video annotation tools available, choosing the right one for your organization requires careful consideration of several factors:
1. Define Your Objectives
Identify what you need from a video annotation tool based on your specific machine learning project. Clarifying your objectives will guide you in selecting the features that best meet your needs.
2. Evaluate Your Budget
Consider the financial implications of different tools. While some video annotation platforms may require significant investment, others offer flexible pricing models suited for various budgets.
3. Assess Scalability
As your project expands, your annotation tool should be able to scale with you. Look for features that support large datasets and an increasing number of users without sacrificing performance.
4. Test Usability
Before committing to a tool, take advantage of free trials or demonstrations. These sessions will provide insight into the user experience and whether the tool aligns with your team's capabilities.
The Future of Video Annotation in Machine Learning
As the demand for high-quality training data increases, video annotation technology continues to advance. Innovations such as automated annotation and integration with artificial intelligence are making the process even more efficient. For instance, using pre-trained models to suggest annotations can significantly reduce the time spent on labeling tasks, allowing human annotators to focus on complex cases that require expert judgment.
Furthermore, the growing integration of virtual reality (VR) and augmented reality (AR) in training datasets signifies an exciting shift. Video annotation tools will likely evolve to incorporate these technologies, providing richer datasets for training machine learning models.
Conclusion
In conclusion, video annotation tools for machine learning are crucial for the success of any project requiring high-quality, labeled video data. With enhanced accuracy, improved development timelines, and a range of applications across various industries, these tools are indispensable for organizations seeking to leverage machine learning effectively.
At KeyLabs.ai, we understand the critical role that video annotation plays in shaping the future of machine learning. Our data annotation platform is designed to empower businesses with efficient, scalable, and user-friendly solutions that meet their unique data needs. As the landscape of machine learning continues to evolve, investing in the right tools will be pivotal in maintaining a competitive edge.
video annotation tool for machine learning